HOW FOUNDATIONAL MODELS REVOLUTIONIZE TIME-SERIES FORECASTING
Time-series forecasting is the practice of using past data to predict future events, imagine if you could forecast the stock market, health, weather, and more with high accuracy and confidence. You could make smarter decisions, optimize your resources, and plan ahead for any scenario. Sounds too good to be true? Enter foundational models, leveraging AI advancements to revolutionize forecasting with unmatched precision and adaptability. This breakthrough signifies a BIG leap forward, promising to enhance our forecasting capabilities and redefine our approach to anticipating the future.
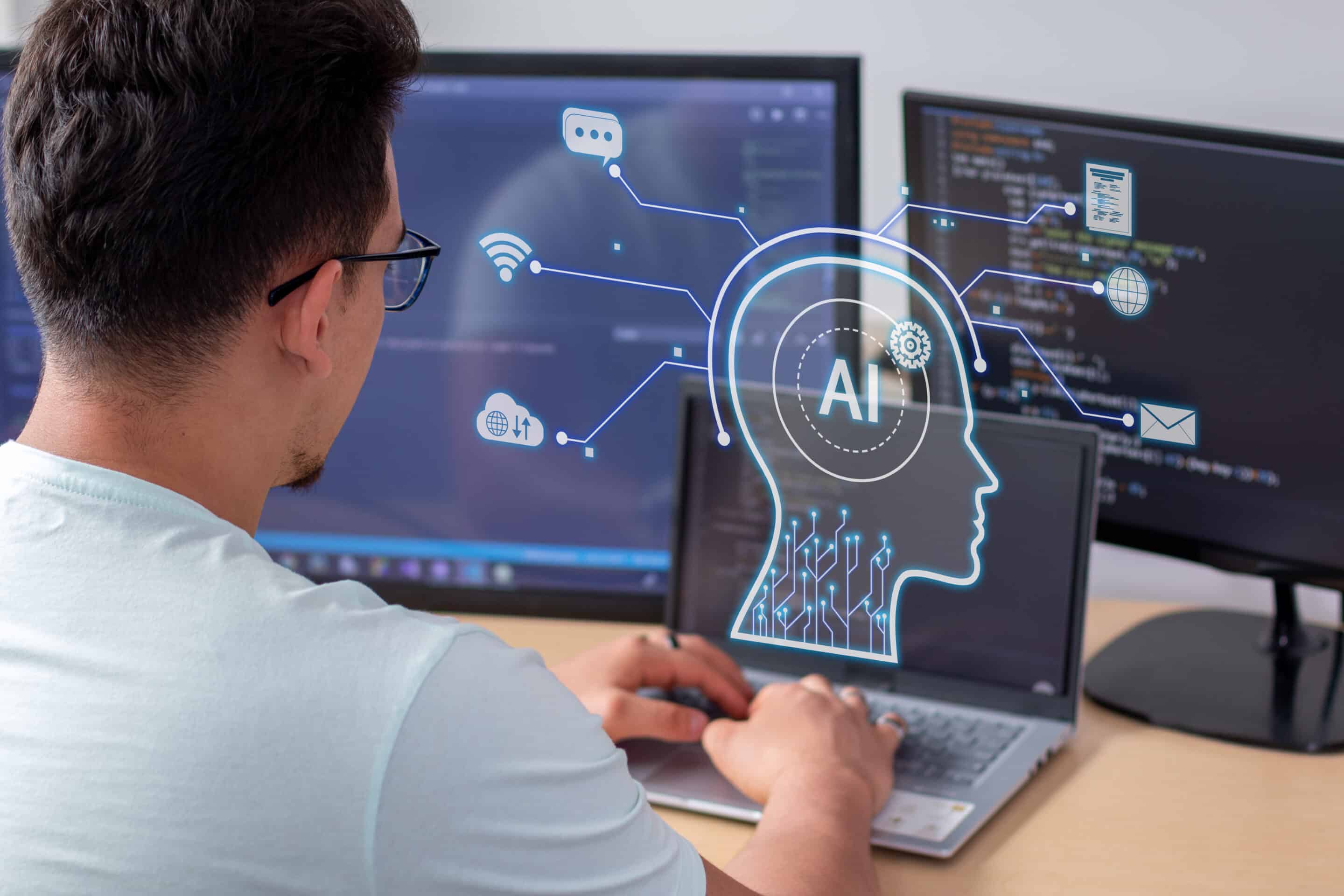
What are foundational models?
Foundational models, GPT-4 for instance, are super-smart AI systems developed by analyzing extensive collections of text from the internet, enabling them to recognize patterns, understand context, and generate predictions with a remarkable level of accuracy. They’re like sponges, soaking up all sorts of knowledge, which helps them get really good at spotting patterns, understanding context, and even making smart guesses. What’s special about them is their flexibility – you can tweak these models to do all sorts of tasks, from generating content to improve customer support. It’s like having a Swiss Army knife for digital tasks.
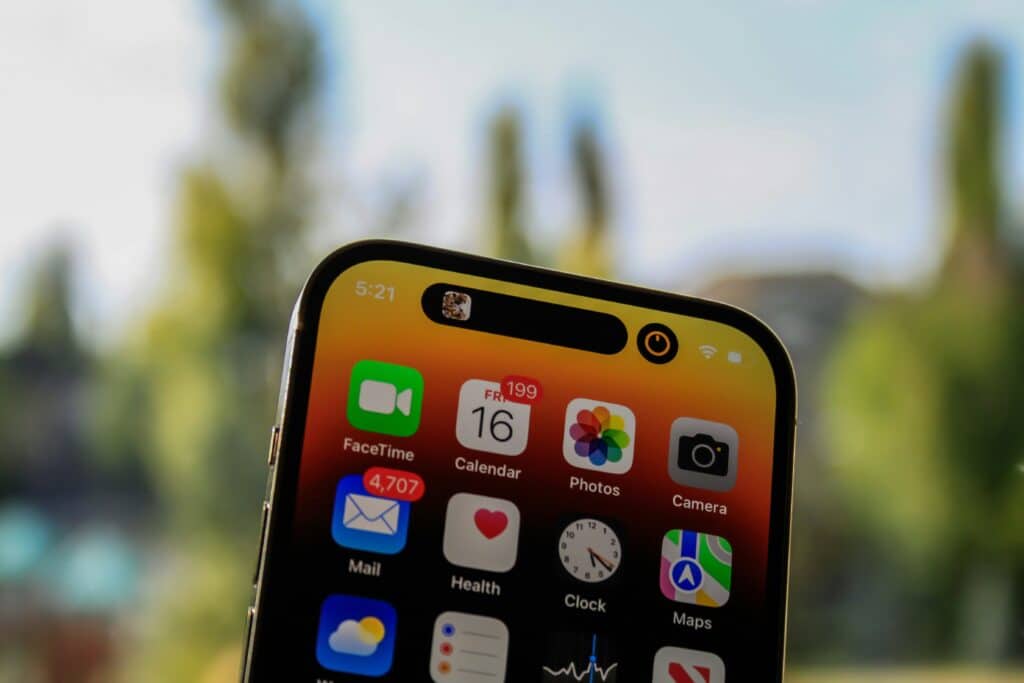
Think of a foundational model as a smartphone. Just as a smartphone can call, take photos, manage emails, and navigate roads, depending on the app you open, a foundational model can write texts, generate images, translate languages, or answer questions, depending on how it’s prompted or what task it’s given. The same device (or model) holds the potential for countless applications, transforming its function with each app (or task) you select, showcasing its flexibility and wide-ranging capabilities in a compact, integrated form.
Time-series forecasting with foundational models
The importance of precise forecasting in sectors such as retail cannot be overstated – it’s integral to inventory management and strategic planning. Foundational models bring unparalleled precision and adaptability to these tasks. An illustrative example of this is Google’s TimesFM, which showcases the practical application of AI to improve forecasting accuracy. However, the true innovation lies in the broader capabilities of foundational models. These models are driving a transformative shift in forecasting, leveraging deep AI insights to offer predictions across various domains, not limited to specific tools or applications.
Addressing the challenges of traditional forecasting
Traditional forecasting techniques have often found it challenging to keep pace with rapid market changes or to analyze complex data comprehensively. Deep learning models, such as Convolutional Neural Networks (CNN) and Long Short-Term Memory (LSTM) networks, have offered a glimmer of hope, but they have been limited by their need for large amounts of historical data and the difficulty in adapting to new data without extensive retraining.
In this context, foundational models stand out by offering a new approach: the capacity for zero-shot learning. This ability to generate accurate forecasts for completely new scenarios without the need for prior training marks a huge step forward. It addresses longstanding limitations of traditional forecasting methods, providing a dynamic and flexible solution to the ever-evolving challenges in predicting future trends.
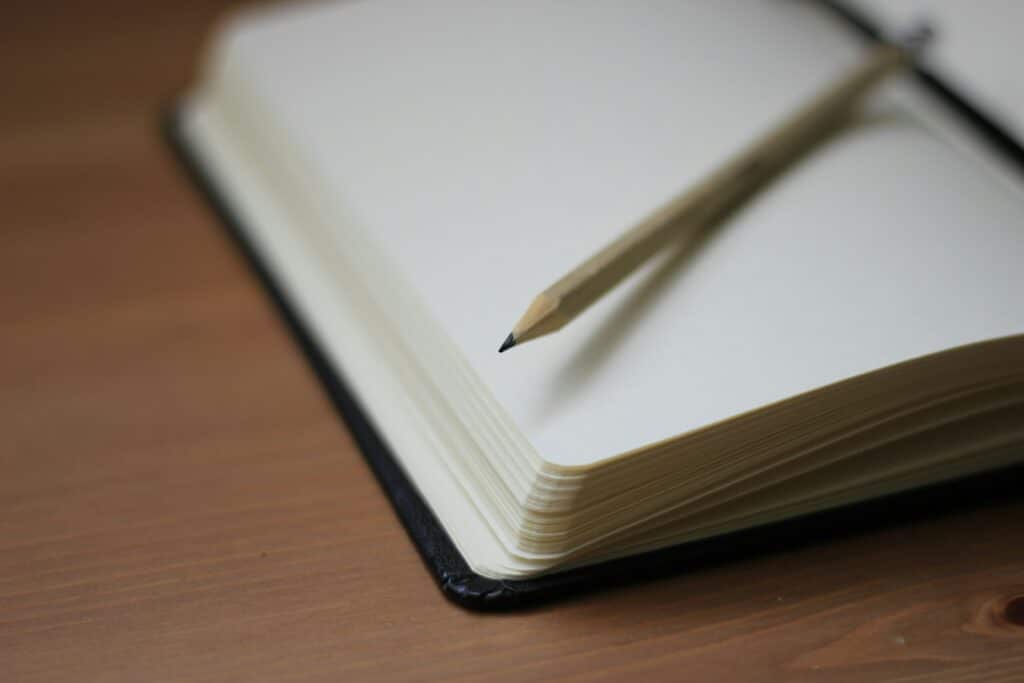
What is Zero-shot learning?
Zero-shot learning is an AI technique that allows a model to accurately handle and make predictions for tasks it has never been trained on before, based on its ability to generalize from related knowledge.
Advantages of foundational models
The profound impact of foundational models stretches across multiple aspects of business operations, heralding a new era of efficiency and strategic insight. By harnessing the predictive power of these AI systems, companies can anticipate demand with greater accuracy, ensuring that supply aligns perfectly with consumer needs. This foresight allows for optimized inventory levels, minimizing both excess stock and shortages, which in turn leads to cost savings and maximized profits.
In addition to operational efficiencies, foundational models play a pivotal role in customer engagement and retention strategies. By predicting consumer behavior trends and preferences, businesses can tailor their offerings and marketing messages to meet the evolving desires of their target audience. This level of personalization enhances the customer experience, fostering loyalty and driving sales.
Looking ahead: the future of forecasting with foundational models
The adoption of foundational models in time-series forecasting signifies a significant transition in the methods businesses use to anticipate and plan for the future. The breakthroughs in natural language processing (NLP), such as those demonstrated by ChatGPT, highlight the broader applicability of these models in various machine learning and artificial intelligence sectors. As these models continue to develop, their ability to impact industries well beyond the scope of forecasting becomes increasingly clear.
Looking forward, the importance of foundational models in areas like sales forecasting is undeniable. Their advanced capabilities offer a robust tool for businesses seeking to refine their predictive accuracy. Moving towards these technologies is becoming less about maintaining competitiveness and more about harnessing the full potential of AI to innovate and lead in their respective fields.
NEWS. INSIGHTS. THOUGHTS.
Budgeting AI: A practical guide for CFOs
Budgeting AI: A practical guide for CFOsEmbracing AI can be a game-changer for businesses looking to improve efficiency and innovate their services. However, the financial aspect of AI adoption can be tricky for many CFOs. This guide provides straightforward steps to...
Create success through smart analysis
Creating success throughsmart analysisManaging a large volume of data makes it essential not only to collect information but also to analyze it in a structured and targeted manner. Building a smart analysis is a powerful method that leads to deeper insights,...
This is self-learning AI
THIS IS SELF-LEARNING AIAI in the food industryWhen you stream a series on Netflix or enjoy a carefully curated playlist on Spotify, sophisticated algorithms work quietly in the background, tailoring your recommendations to your preferences. This technology, known as...