This is self-learning AI
When you stream a series on Netflix or enjoy a carefully curated playlist on Spotify, sophisticated algorithms work quietly in the background, tailoring your recommendations to your preferences. This technology, known as self-learning artificial intelligence, is exactly what it sounds like—an AI system capable of learning and improving on its own without further human intervention after initial programming. These systems adapt to new situations, learn from past experiences, and develop strategies to solve problems. Read on to discover more about this technology and how self-learning AI can benefit you and your business.
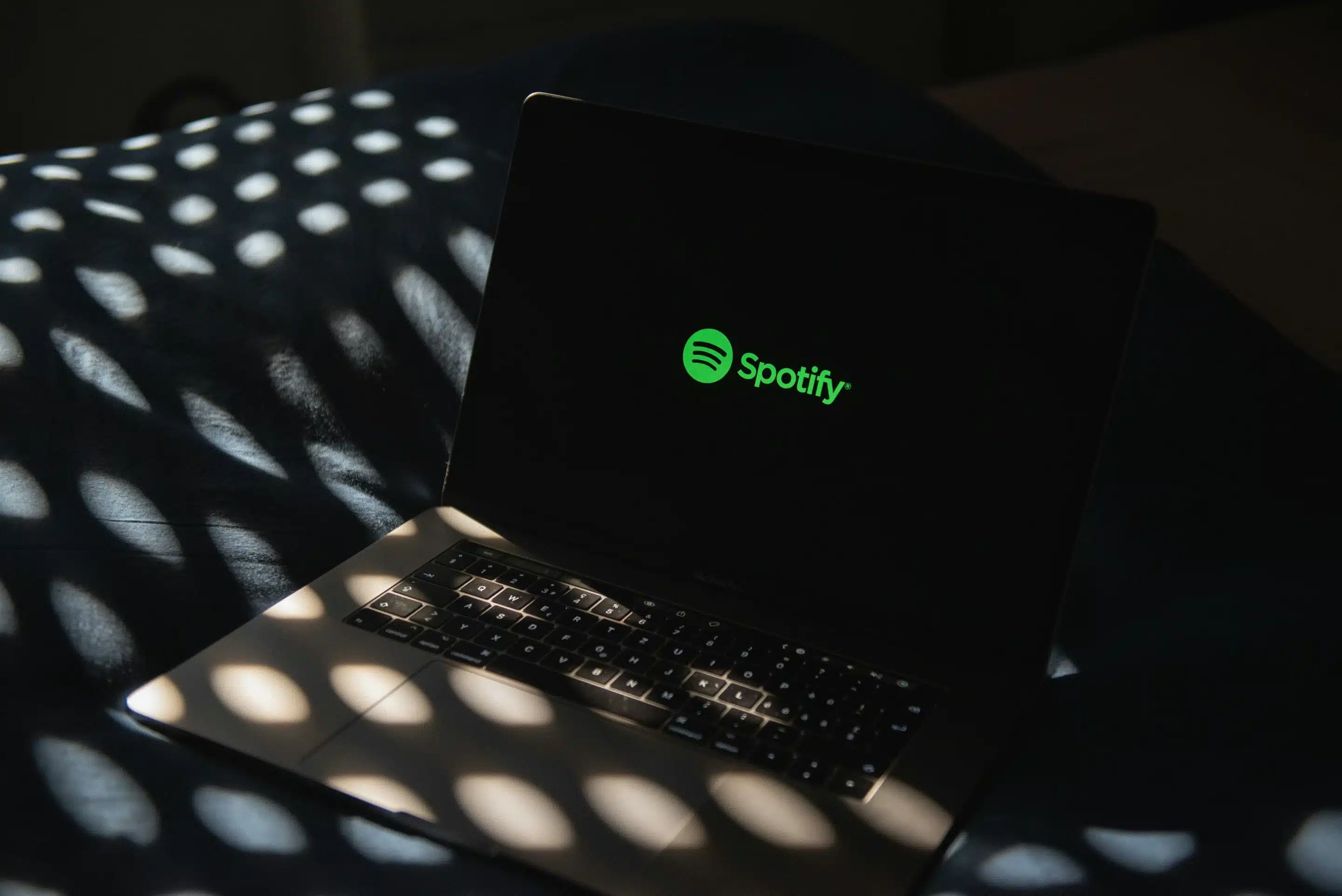
to recommend relevant content.
The benefits of self-learning AI
Self-learning AI excels in identifying patterns and deriving insights from data, even in projects with limited initial data points. This capability is crucial for quickly understanding and improving new products based on minimal testing.
Additionally, self-learning AI is highly adaptable. When new data emerges, either from its own testing or when the AI is asked to do something new, it can relearn and adapt without starting from scratch. This saves time and effort as the same AI can be utilized across multiple projects.
In short, self-learning AI makes it easier and faster to work on complex problems.. It’s like having a smart colleague who learns on the fly and is always ready for the next challenge.
How does self-learning AI work?
To fully appreciate the potential and mechanisms behind self-learning AI, let’s examine its three fundamental pillars: Machine Learning, Deep Learning, and Reinforcement Learning.
Machine Learning (ML) Machine Learning is the core technology behind self-learning AI. ML models are trained on large datasets to identify patterns and make predictions. As more data is collected, these models can be updated to enhance their accuracy.
Deep Learning A subset of ML, Deep Learning utilizes neural networks with multiple layers (hence “deep”) to simulate human decision-making processes. Deep Learning has revolutionized fields such as image and speech recognition.
Reinforcement Learning (RL) Reinforcement Learning is a type of ML where an “agent” learns to make decisions by performing actions in an environment to maximize some form of reward. RL has been used to develop systems that can outperform humans in complex games like chess and Go, without being pre-programmed with specific strategies.
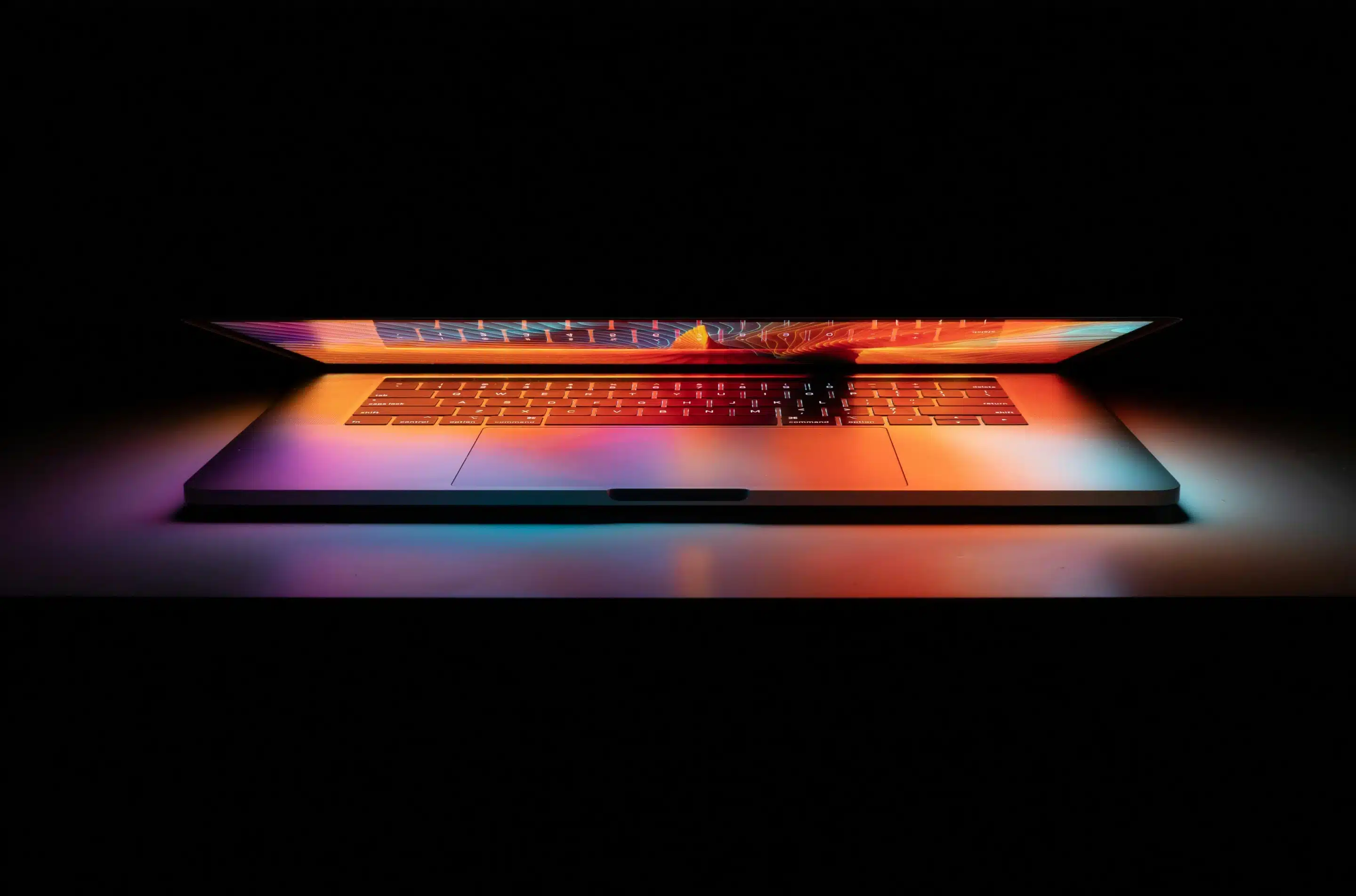
The difference between traditional AI and self-learning AI
Traditional AI, or conventional artificial intelligence, typically refers to systems programmed to perform specific tasks based on predetermined rules and algorithms. These systems are often limited to what they were originally designed for and lack the ability to learn or adapt to new data or situations without human intervention.
Examples of traditional AI
Expert Systems: These are computer programs that mimic human expertise in specific areas to provide advice or make decisions. They use a database of specialized knowledge and a set of rules to analyze information and solve problems, similar to how Google’s spelling suggestions correct misspellings in the search box.
Rule-Based Systems: These AI systems use a series of pre-programmed rules and conditions to analyze data and make decisions or recommendations, much like how an antivirus program uses predefined rules to identify and manage potential threats on a computer.
Self-learning AI, on the other hand, are systems that learn from data and experiences to improve their performance over time without being explicitly programmed for each new task.
Examples of self-learning AI
Language Translation: Self-learning AI is used to enhance language translation services by continuously learning from translation data to produce more accurate and natural translations over time. Examples include Google Translate and DeepL.
Recommendation Systems: Platforms like Netflix, Spotify, and Amazon use self-learning AI to analyze user preferences and behaviors to recommend content, music, or products that are most relevant to each individual user.
Autonomous Vehicles: Self-learning AI is employed in autonomous vehicles to continually improve their ability to detect and respond to traffic conditions and surrounding obstacles. Companies like Tesla, Waymo, and Uber are leaders in developing this technology.
Overall, the main difference between traditional AI and self-learning AI is the latter’s ability to learn and adapt. Traditional AI depends on explicit instructions and cannot learn from new data, while self-learning AI leverages machine learning and related technologies to continuously improve and adapt based on new information.
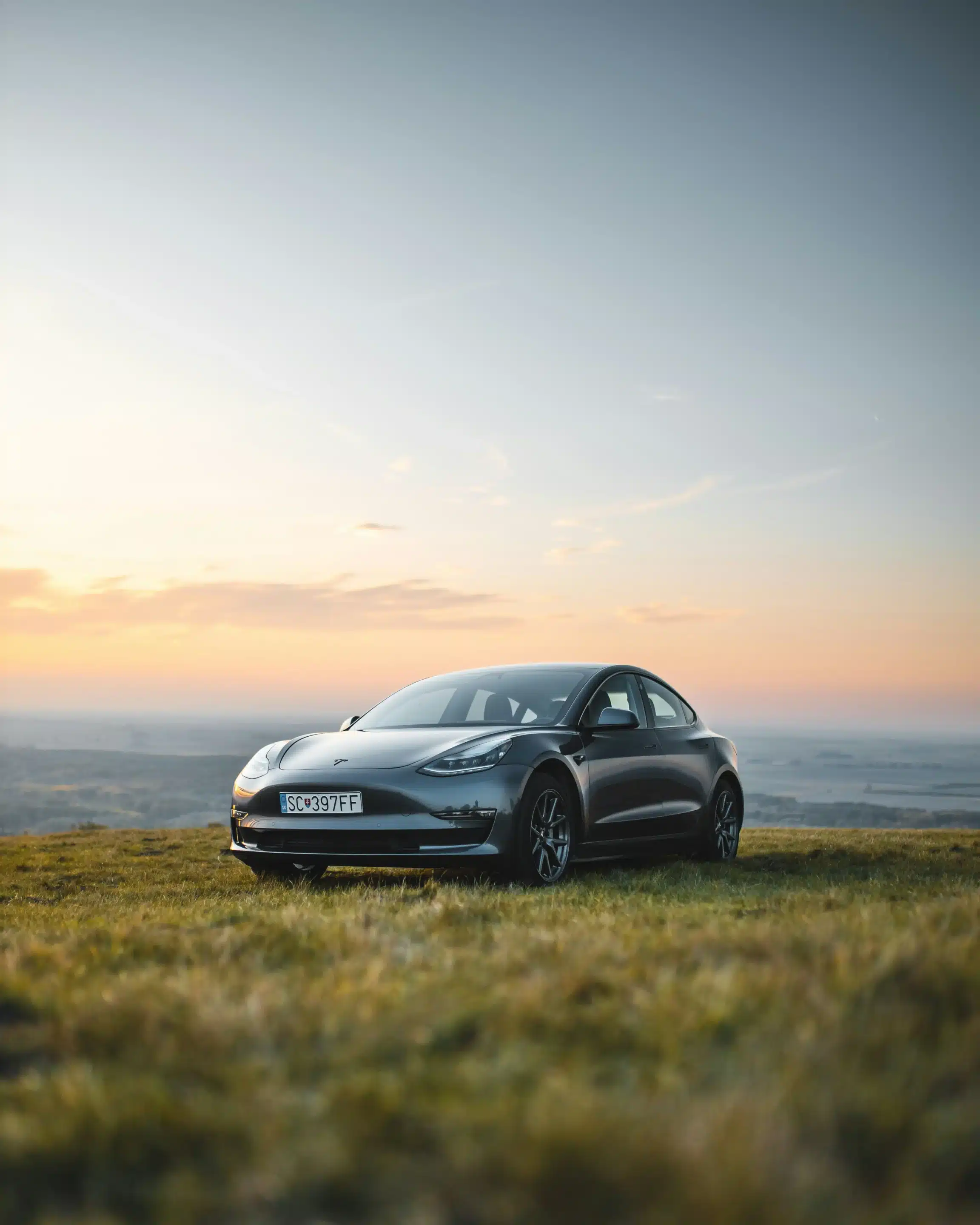
self-driving technology using AI.
Leveraging self-learning AI in your business
Now that we’ve discussed the concepts and potential of self-learning AI, it’s important to consider how you can benefit from this technology within your business. If you work in a food production company, for example, there are several ways to integrate self-learning AI into your operations to enhance efficiency and innovation.
Quality control
By using AI to analyze images or sensor data from the production line, you can quickly and accurately detect any defects or anomalies in the products. This helps reduce waste and ensures that only high-quality products reach the market.
Optimizing production processes
Analyzing production data allows you to identify inefficiencies or bottlenecks and make adjustments to improve productivity and reduce costs.
Demand forecasting and inventory management
By analyzing historical sales data and external factors such as weather conditions or promotions, you can make more accurate forecasts and minimize the risk of over- or under-production.
News. Insights. Thoughts.
Elvenite upgrades Snellman to M3 Cloud and begins collaboration in the Finnish market
Elvenite upgrades Snellman to M3 Cloud and begins collaboration in the Finnish market We are taking an important step into the Finnish market by upgrading Snellman's ERP system to M3 Cloud. The upgrade marks the beginning of an exciting partnership that will provide...
Elvenite sustainability report 2023
Elvenite sustainability report 2023We are proud to present Elvenite's sustainability report for 2023! Throughout the year, we have focused on improving resource utilization and reducing waste in the food industry using solutions such as AI-driven seed processing and...
AI assistants at the forefront: Google’s Project Astra
AI assistants at the forefront: Google's Project AstraGoogle's Project Astra are redefining how we interact with technology both in personal and professional contexts. These innovations are not only enhancing our interactions with AI but also deeply integrating AI...