Reducing waste in In-Store Bake-Off (with Open Data!)
Data powers technologies like artificial intelligence, business insights, and predictive analytics, much like oil powers machines. Just as oil becomes more valuable when refined, data also gains value when processed. However, unlike oil, data becomes more valuable the more it is used. When different datasets are combined, they can create magical insights that open up entirely new worlds of possibilities.
What is open data?
Open data is information that anyone can use freely, for practically any purpose, without restrictions such as costs or licenses. Essentially, it’s data that can be accessed, used, and distributed by anyone. Open data can be of various types, including financial, statistical, geographic, or meteorological data. When used smartly, open data can foster innovation, drive revenue and economic growth, accelerate development, and much more.
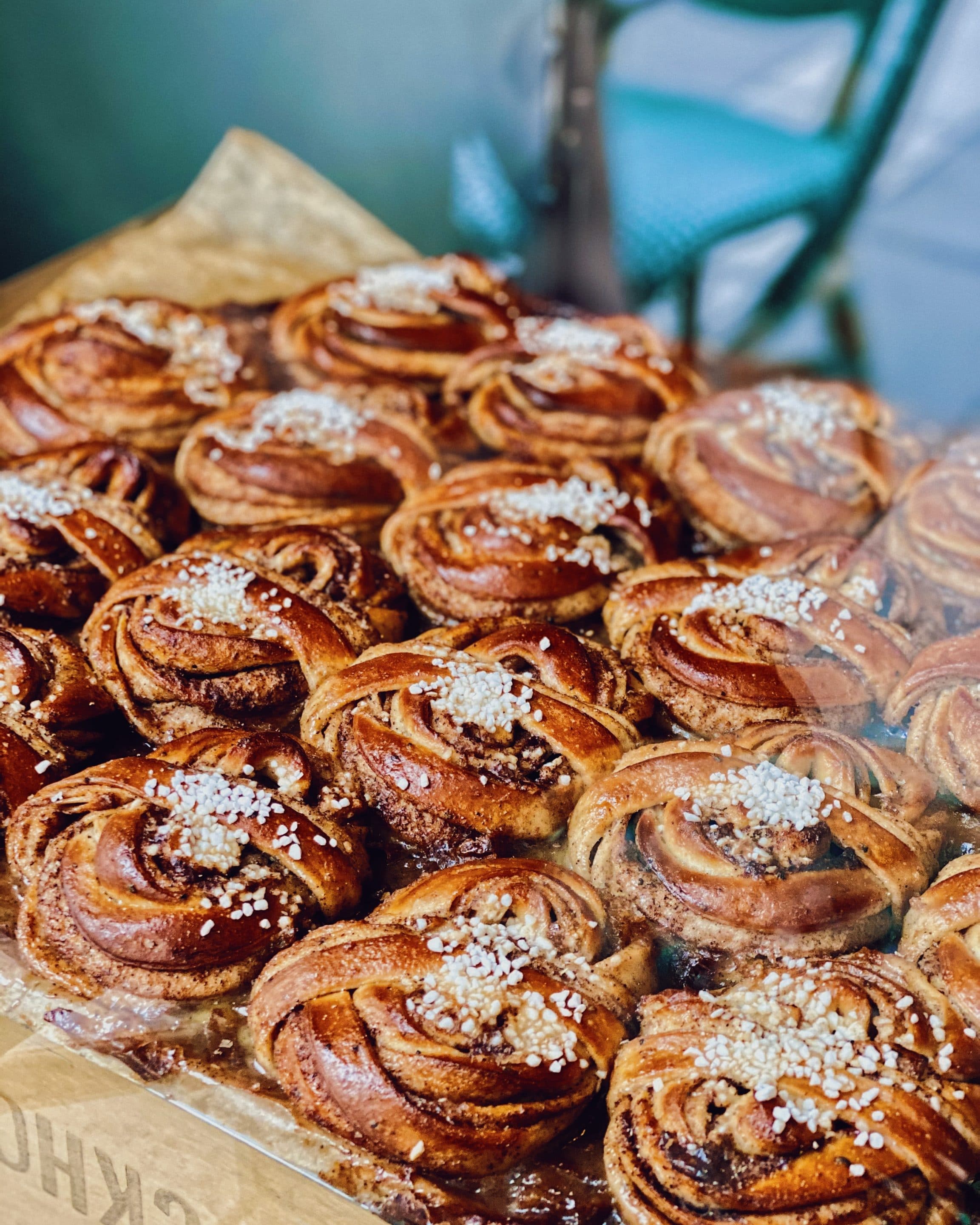
Where does open data come from?
The most common distributors of open data are public sector entities. In Sweden, the government has tasked the Agency for Digital Government (Digg) with promoting the ability of public actors to share and utilize data for research and innovation. Increasingly, private sector organizations are also recognizing the potential of using and sharing both external and internal data.
Open data – effective for reducing waste
To illustrate the practical application of open data, let’s share an example where we used open data to reduce waste. In grocery stores, in-store baked bread (known as bake-off) is a significant driver of additional sales, but it can also lead to substantial waste.
In our example, a grocery chain sold bake-off products worth 50 million SEK per year, with waste accounting for about 10% of sales. The problem seemed to be that they were not offering what customers wanted, or at least not at the right time. In other words, there was a lack of an optimization model to efficiently determine what should be baked and when during the day to minimize waste. So, we started a project to see if we could optimize in-store baking through data analysis.
The customer had never considered what could sell well based on other parameters; they simply stopped baking what wasn’t selling well at the moment. To solve the problem, we needed to understand what factors influence bake-off sales. We began by analyzing the large datasets that the customer already had and looked at the intuitive factors first:
- Sales data from different days and times of the day
- Campaign data
- Cross-promotional sales (e.g., coffee on the same receipt as bake-off items; has bake-off sales increased during coffee promotions?)
- Analysis of patterns on different days such as Cinnamon Bun Day, payday, seasonal variations, etc.
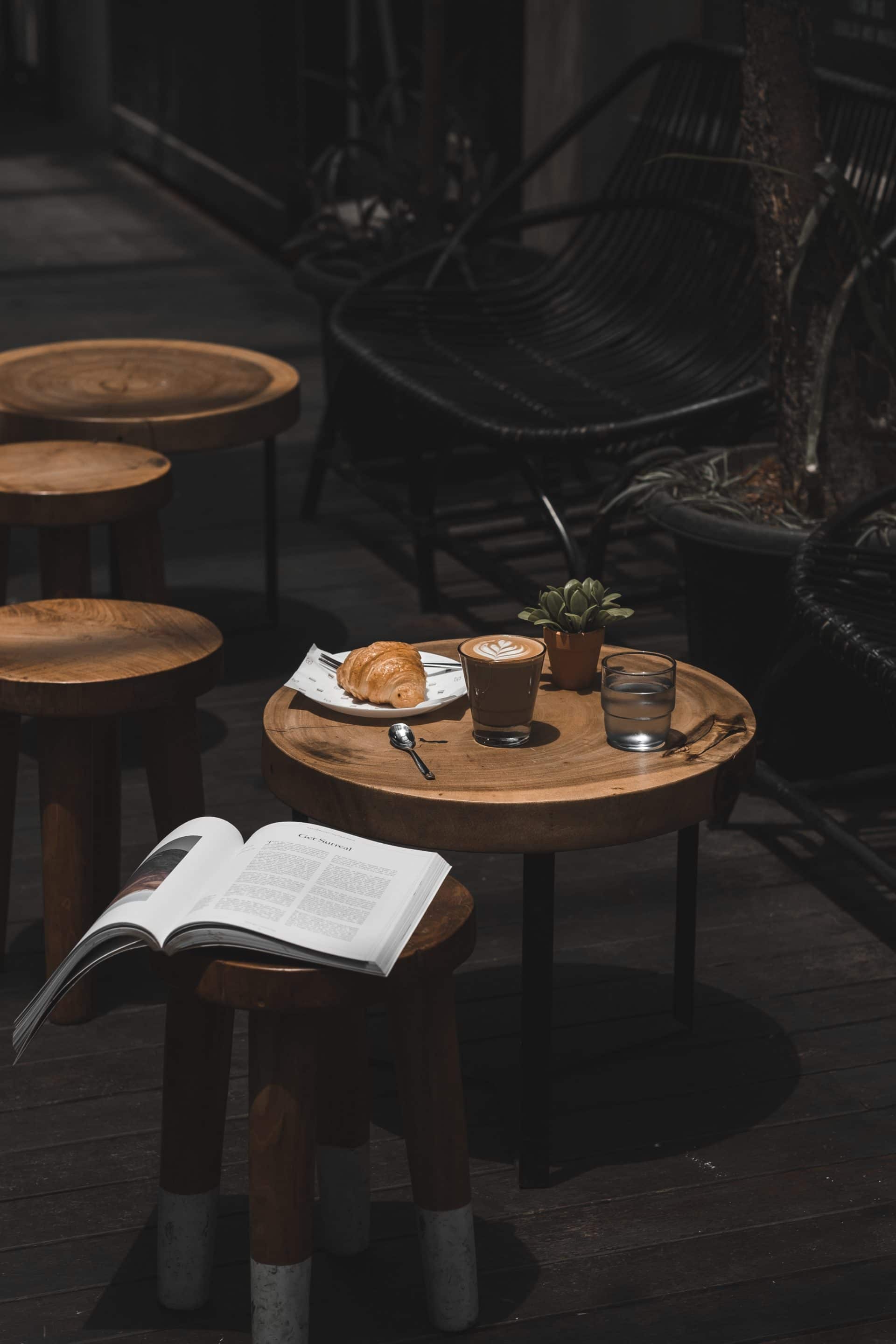
To better understand…
…what was important for a specific grocery store, we then added a large amount of open data. We weighted the sales against demographic data from DeSo-data (Demographic Statistical Areas) in each store’s catchment area. This allowed us to identify possible patterns among the residents, such as:
- Different ages
- Family circumstances
- Socioeconomic background
An example of a pattern might be older people buying bake-off items early in the morning, or younger people buying more donuts than cinnamon rolls.
We also weighted the existing sales data against Google Maps APIs to get more information about the surrounding offerings in each DeSo area. For example:
- Are there popular cafés or restaurants in the area?
- Is there a very good café in the area? If so, perhaps the store shouldn’t sell bake-off cinnamon buns.
- Workplaces and schools – are there patterns indicating that workplaces buy Friday treats? Do schools generate sales during lunch breaks?
The area’s demographics were analyzed and compared with the store’s own data to identify any sales patterns. This data analysis created an understanding of what actually influenced both the quantity and choice of baked goods sold – and what didn’t.
We then built an optimization model that the store could use to determine what, when, and how to bake throughout the day.
As a result, the chain, which previously always baked full trays of everything because “that’s how it’s always been done,” started baking “mixed trays” in stores, significantly reducing waste.
Open data, Open doors
This is just one example of how open data and the combination of different datasets can be used to improve or develop products and services. As the amount of open data increases, the possibilities become endless!
News. Insights. Thoughts.
Elvenite ranked as one of the best places to work in the IT industry in 2024
Elvenite ranked as one of the IT industry's Best Workplaces 2024 Elvenite has been named one of Sweden's Best IT Workplaces for 2024, a prestigious award from Great Place To Work®. Elvenite is ranked 15th out of 25, celebrating our outstanding workplace culture and...
When milk production flows steadily, year-round
Ensuring steady milk production all year round with AIDid you know that milk production fluctuates significantly throughout the year? While we, as consumers, buy milk at the same pace regardless of the season, dairies like Valio face major challenges. During the...
How we use word embeddings and AI: from seating arrangements to data analysis
How we use word embeddings and AI: from seating arrangements to data analysisCreating the perfect seating arrangement is a challenge we can all relate to, whether it’s a private party or a large corporate event. Should I seat Anna next to Henrik, or will she be...