Creating success through
smart analysis
Managing a large volume of data makes it essential not only to collect information but also to analyze it in a structured and targeted manner. Building a smart analysis is a powerful method that leads to deeper insights, well-founded data-driven decisions, and therefore competitive advantages.
Here, we provide you with concrete tips in 4 steps to help you build a smarter analysis from the ground up, enabling you to maximize the value of your data insights and achieve desired results.
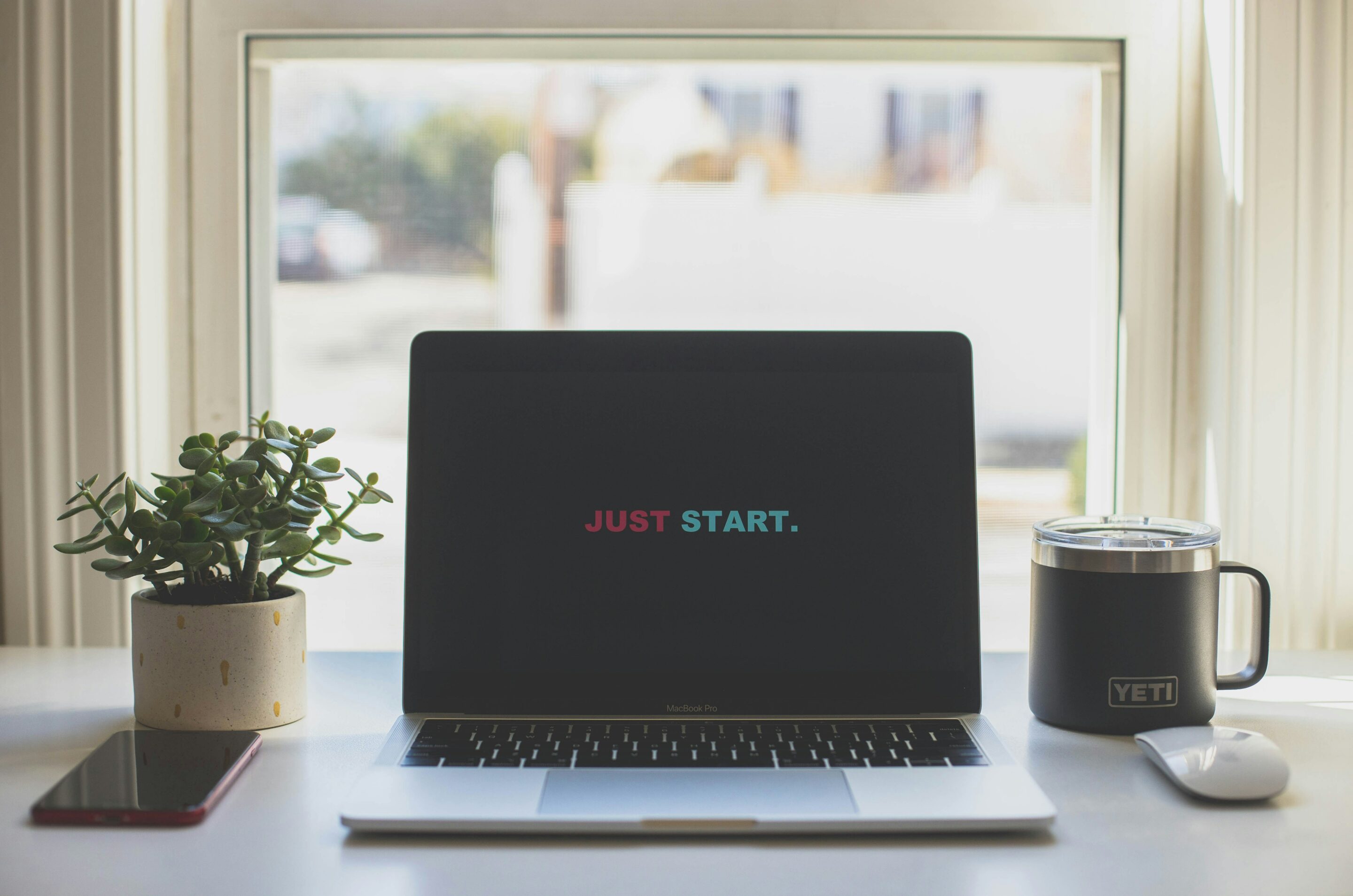
1. Define your objectives and the questions you want to answer.
Prior to diving into your data, it’s essential to have a clear strategy and well-defined goals. Defining your goals and the questions you aim to address represents the initial step toward a successful analysis. By understanding what you are trying to achieve, you can focus your analysis more effectively and thus achieve the best possible results.
Questions such as ‘What is the purpose of the analysis? and “What insights are we trying to uncover?” are important to ask in order to target your efforts and resources effectively. Clearly defined goals create a clear direction for your analysis.
2. Make your analysis clear and efficient
Clarity is key to effective analysis. To achieve a clear analysis, you need to use a structured approach that ensures you don’t miss any important insights. A good example of a structured approach is the Top-Down method:
I. Start with an overarching perspective and identify the main results. Getting an overview of your data is crucial to understanding the major trends and patterns. Define the most important KPIs and dimensions for your analysis objective.
II. Expand, filter and drill down into the data to get a more detailed understanding. After creating an analysis with the main results, you also want to see the underlying causes and patterns. By enabling different time, filtering and dimensional perspectives, you can gain a more detailed understanding of your data and identify drivers and trends.
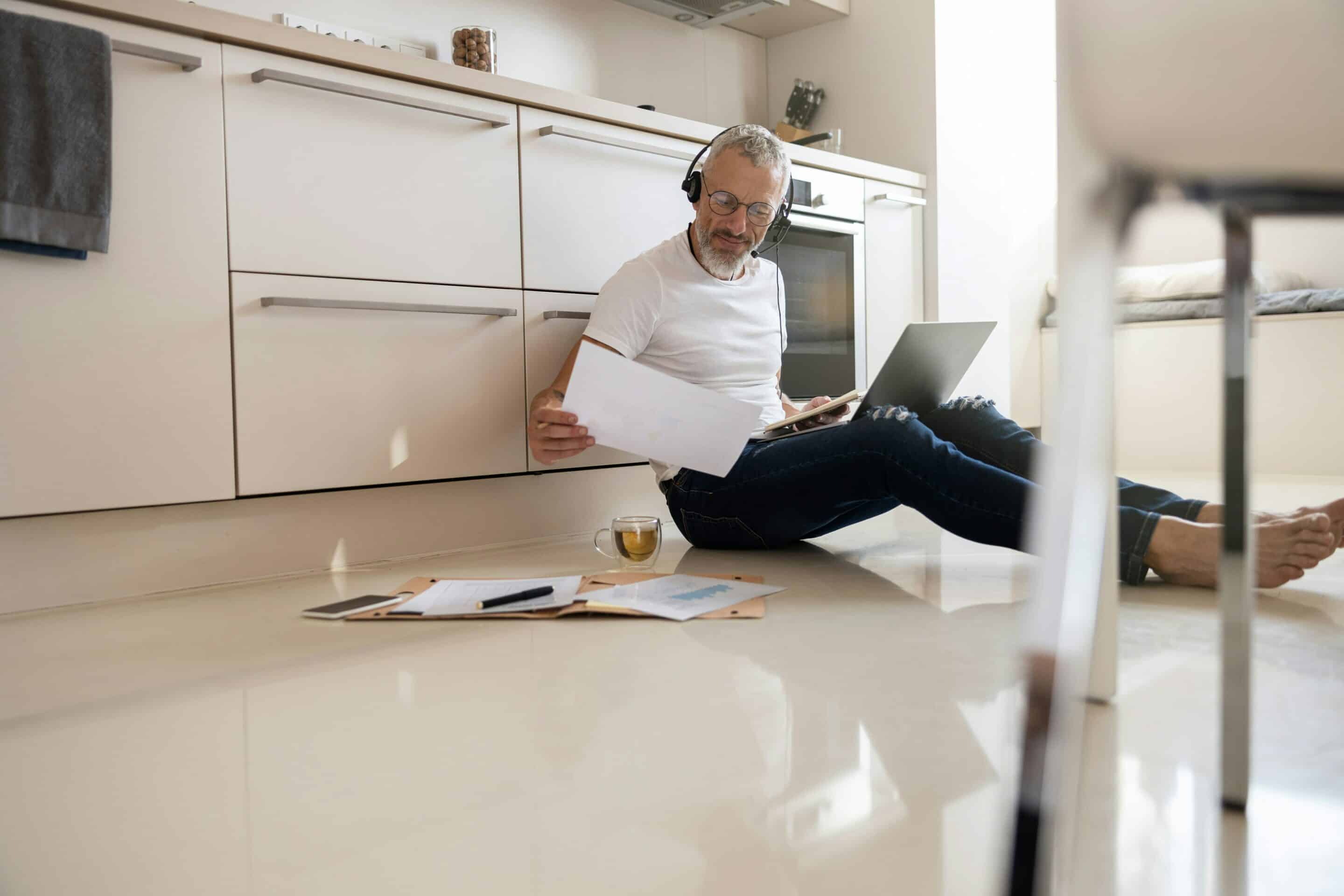
3. Tell a story with your data!
Leveraging storytelling techniques becomes an effective approach for communicating insights with large datasets. Structure your analysis by sharing the causes, patterns and insights behind the results using dimensions and KPIs.
Follow a sequential order and prepare yourself by asking relevant follow-up questions that are likely to arise during the presentation of the analysis. Which dimensions, KPIs and time horizons will be relevant?
Answer the questions and pose the same question for each step. When presenting your analysis, start by explaining the overall result and then, step by step, dissect the analysis to answer any follow-up questions and interesting occurrences.
4. Conclude by drawing conclusions and recommendations based on your analysis.
Ultimately, drawing conclusions and offering recommendations based on your analysis are essential steps to making your results meaningful and actionable.
Nyheter. Insikter. Omtanke.
Elvenite ranked as one of the best places to work in the IT industry in 2024
Elvenite ranked as one of the IT industry's Best Workplaces 2024 Elvenite has been named one of Sweden's Best IT Workplaces for 2024, a prestigious award from Great Place To Work®. Elvenite is ranked 15th out of 25, celebrating our outstanding workplace culture and...
When milk production flows steadily, year-round
Ensuring steady milk production all year round with AIDid you know that milk production fluctuates significantly throughout the year? While we, as consumers, buy milk at the same pace regardless of the season, dairies like Valio face major challenges. During the...
How we use word embeddings and AI: from seating arrangements to data analysis
How we use word embeddings and AI: from seating arrangements to data analysisCreating the perfect seating arrangement is a challenge we can all relate to, whether it’s a private party or a large corporate event. Should I seat Anna next to Henrik, or will she be...